Why AI’s future success will be determined by entrenched guilds
The Blue Check Commentariat (likely to be shrinking dramatically on April Fool’s Day today) has been voluminously twittering on the labor implications of AI products like ChatGPT. Will doctors and lawyers be made obsolete? Is customer support work dead? Will there be a marketer left in the building?
Will the last person still employed please turn off the light? (Damn, AI already does that too, doesn’t it?)
I sketched my theory on AI’s implications for the creative class in “ChatGPT as garrulous guerrilla” (I think the creative class is by and large doomed), and since then, two other prominent analyses have been published. One was by Goldman Sachs, which headlined that 300 million jobs could be affected by AI, while another one came from OpenAI, OpenResearch and Penn researchers, who concluded that “around 80% of the U.S. workforce could have at least 10% of their work tasks affected by the introduction of LLMs [Large Language Models].”
These analyses are illuminating, but they evade how “work” actually works: the questions of who gets to do which work and how they get to do it. The answers to these questions are rarely determined by emerging technologies or even at times economics, but are rather determined by the power of individual guilds and professions to control monopolies over work in a field.
While it’s never mentioned in technology circles, Andrew Abbott’s The System of Professions: An Essay on the Division of Expert Labor remains one of the most important works in the canon of economic sociology (and currently sits at just shy of 19,000 citations according to Google Scholar). Deeply influential, Abbott argues that professions fight one another for “jurisdiction” over a field of work, and it is this rivalry and its interconnected social relations that determine how work actually gets done and by whom.
Take the act of prescribing pharmaceutical drugs. It’s an activity central to allopathic medical doctors, a profession represented by the American Medical Association and which tends to its monopoly through state medical licensing boards, strict and extensive education requirements, and strong impediments on the immigration of foreign doctors into the United States. This activity could theoretically be done by anyone, but doctors have a monopoly on the work.
Adjacent to doctors is the nursing profession, which has its own licensing and curriculum requirements that match a different set of duties. Nurses can watch doctors prescribe medications for decades, but they aren’t authorized to write those scripts themselves. In theory, there’s a clear boundary of activities between the two professions.
Compared to nurses though, doctors are extremely expensive. Thanks to the AMA’s monopolization, the supply of physicians is not growing nearly fast enough to match the massively rising demand from patients. The combination of huge economic disparity and acute demand provided an opportunity for nursing to gain new jurisdiction over an activity excluded to the profession in the past, in the form of nurse practitioners (NPs).
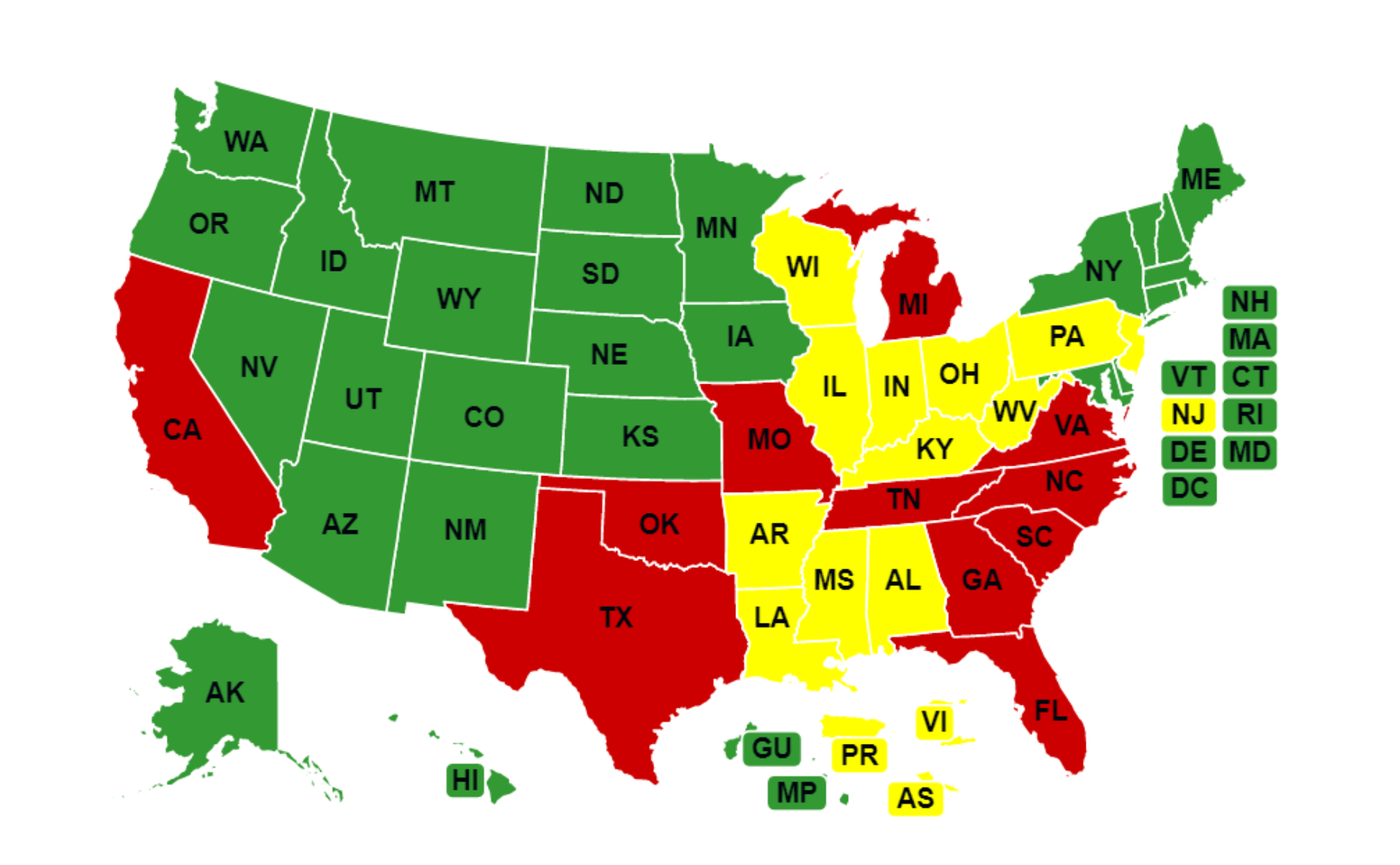
Nurse practitioners — depending on a state’s laws — have the ability to provide typical medical care including referring a patient to diagnostics and labs as well as prescribing drugs. NPs are a relatively new hybrid profession, a sort of midpoint between the nursing and medical professions, and its rise has led to the development of companies like MinuteClinic that take advantage of these new professional fault lines.
It’s been a tough fight for the AMA, and one that it has lost in many states. Nonetheless, the organization remains resolutely opposed to the development of NPs. As it notes on its advocacy website, “For over 30 years, the AMA’s state and federal advocacy efforts have safeguarded the practice of medicine by opposing nurse practitioner (NP) and other nonphysician professional attempts to inappropriately expand their scope of practice. […] Since 2019, the AMA secured over 100 state legislative victories stopping inappropriate scope expansions of nonphysicians. This work was done in strong collaboration with state medical and national specialty societies.”
Technology has had little effect on these fights over control of the Rx pad. The Covid-19 pandemic triggered a massive expansion of virtual-care appointments, but those appointments must usually be staffed by the same licensed professional as was required before for in-office visits. Software might have replaced some mundane tasks of doctors — but doctors determine what technologies doctors are allowed to use.
The professions gets a voice, and on the whole, has voiced no to most automation. The AMA doesn’t even call AI “artificial intelligence”. Per its notes, “The AMA House of Delegates uses the term augmented intelligence (AI) as a conceptualization of artificial intelligence that focuses on AI’s assistive role, emphasizing that its design enhances human intelligence rather than replaces it” (emphasis added). Computers aren’t displacing humans here.
Depending on how you count, there are hundreds or even thousands of professions, ranging from doctors and lawyers to architects and civil engineers. In each case, the profession dictates the standard of work and the kinds of tools allowed to be used in their trade.
Which brings me back to AI and employment: there are clearly well-organized organizations that protect the jurisdiction of these professions, just as Abbott theorizes. The AMA has fended off attempts to democratize medicine for more than a century against Congress, the President, fundamental economics and citizen demands (for a lengthy history, see Peter A. Swenson’s Disorder: A History of Reform, Reaction, and Money in American Medicine). The question of AI’s utility in these professions isn’t whether it is competent enough against a well-trained professional, it’s whether that well-trained professional will vote to allow it to replace themselves in the process.
Thus, who will advocate for AI against extremely well-organized and entrenched professions that have the law protecting their domain?
Because let’s be clear: the professional quality of AI has already surpassed at least some working professionals, and will likely surpass the vast majority of them in the years ahead. My (former) CPA (a profession licensed and protected by law) mangled all of my taxes one year by getting nearly every number on my 1040 an order of magnitude wrong. My (former) lawyer (protected by a bar association) once gave such alarmingly bad advice that a quick Google search and a second opinion quickly got us back to legal reality. My (former) doctor (protected by medical licensing) once prescribed the complete wrong drug for me, while the pharmacist (licensed and protected by law) then changed it to an accidentally higher dose. Oops.
ChatGPT, as plenty of people (including Gary Marcus on the “Securities” podcast) have pointed out, has no concept of truth. It takes input and attempts to output the text that makes the most sense given the context it’s offered. It’s not a trained professional with schooling, examinations, licensing, and continuing education requirements. It plops out a word salad given whatever you tell it.
But, so many professionals today lack the time to do their work properly. CPAs give a once-over of a tax document to try to make their annual quotas, while doctors get 15 minutes to take a patient history, debate a solution, and drop the prescription orders into the EMR system. Lawyers on the other hand can bill by the hour, but then obviously face pressure from their clients to limit expenses (and thus the quality of legal advice).
Which performs better work on average? The tired and timed professional or the AI bot? I might dub this question the Professional Turing Test: can you discern whether a human professional or an AI substitute is performing better? If given a human diagnosis or an AI diagnosis, which one would you trust more? The crossover is already here in some cases, and the proportion is only set to increase.
All professions will aggressively fight off AI, in much the way that all professions have fought efforts to open up occupational licensing across the country. Those battles are almost always won by professionals thanks to political mobilization bias: the people most affected are the professionals themselves, who are heavily motivated to protect their labor monopoly compared to the common voter who isn’t picketing in front of the governor’s office demanding more automation at the doctor’s office.
I eluded to it a bit earlier in the context of NPs, but the only hope for AI given Abbott’s theory is to empower a new group of professionals who want to compete with existing professionals for the same jurisdiction. The future won’t be about AI-augmented incumbents, but rather AI-empowered disrupters who want to displace the incumbent professions and force them out of their entrenched jurisdictions.
The upshot is that the United States has a decentralized governance system around licensing that ensures that at least some states will be the first to jump into an AI-driven world. The downside is that AI’s progress in the decade ahead will stall far earlier and last far longer than many analyses predict.
“Securities” Podcast: “Smell can be art, and it also can be science”: AI/ML and digital olfaction

We perceive the world through our senses, yet while scientists have answered fundamental questions about color and audio, there remains a huge gap when it comes to smell. Given how much more complex and higher dimensional it is, smell is an extraordinarily hard sense to capture, a problem which sits at the open frontiers of neuroscience and information theory. Now after many decades of discovery, the tooling and understanding has finally developed to begin to map, analyze and ultimately transmit smell.
Joining me this week on the podcast is Alex Wiltschko, CEO and founder of Osmo, a Lux-backed company organized to give computers a sense of smell. He’s dedicated his life (from collecting and smelling bottles of perfume in grade school to his neuroscience PhD) to understanding this critical human sense and progressing the future of the field. We talk about a massive range of topics on the neuroscience of smell and why the field’s open frontier questions are finally starting to become answered by researchers.
Lux Recommends
- One delightful read this week from Josh Wolfe and his enduring goal of overthrowing the con of tiramisu via the Financial Times in “Everything I, an Italian, thought I knew about Italian food is wrong.” “[Alberto Grandi’s] research suggests that the first fully fledged restaurant exclusively serving pizza opened not in Italy but in New York in 1911. ‘For my father in the 1970s [in Italy], pizza was just as exotic as sushi is for us today,’ he adds.”
- Our scientist-in-residence Sam Arbesman recommends Virginia Heffernan’s piece in Wired on “I Saw the Face of God in a Semiconductor Factory.” "For a company to substantially sustain not just a vast economic sector but also the world’s democratic alliances would seem to be a heroic enterprise, no?”
- “Securities” reader Tess van Stekelenburg notes that protein design AI model RF Diffusion has now been made open source by the Baker Lab at the University of Washington.
- Grace Isford recommends a new research paper by American and Korean scientists titled “CHAMPAGNE: Learning Real-world Conversation from Large-Scale Web Videos”. “… when fine-tuned, [the model] achieves state-of-the-art results on four vision-language tasks focused on real-world conversations."
- Ina Deljkic was entranced by “Elusive ‘Einstein’ Solves a Longstanding Math Problem”. “In less poetic terms, an einstein is an ‘aperiodic monotile,’ a shape that tiles a plane, or an infinite two-dimensional flat surface, but only in a nonrepeating pattern. (The term ‘einstein’ comes from the German ‘ein stein,’ or ‘one stone’ — more loosely, ‘one tile’ or ‘one shape.’)”
- I’ll point out that other countries aren’t standing still when it comes to chip competition (and spare us all another column on the damn CHIPS Act). Korea’s legislature this week passed a “K-Chips Act” to out-subsidize American semi fabs. How high will the subsidies go?
- Finally, Grace, whose family history tracks back to Iceland (and who cooks the traditional Icelandic cake vínarterta although has never offered me a slice), recommends OpenAI’s post about how GPT-4 is helping the country preserve its language. “Prior to [Reinforcement Learning from Human Feedback], the process of fine-tuning a model was labor and data-intensive. Þorsteinsson’s team attempted to fine-tune a GPT-3 model with 300,000 Icelandic language examples, but the results were disappointing.”
That’s it, folks. Have questions, comments, or ideas? This newsletter is sent from my email, so you can just click reply.