Will the winners of AI center on more or less compute?
Both kids and adults occasionally imagine, “What would I do if I was a millionaire?” But these are unequal times — the world’s richest man is now valued at $426 billion by Forbes while the president acquired billions in paper wealth with a memecoin cash grab this week — and so we need to revise the game upwards. “If you had $500 billion, what would you do?”
Apparently, the answer is to buy a data center. A lot of them. All of them.
OpenAI’s Sam Altman announced The Stargate Project earlier this week, a new venture that will spend upwards of $500 billion on data centers and compute for next-generation AI models. $100 billion of that spend will be deployed in the next 12 months. Funding for the project comes from Japan’s SoftBank Group (whose chairman Masayoshi Son will also chair Stargate), Oracle and MGX of the United Arab Emirates.
Beyond getting into an inside-baseball spat with Elon Musk, Altman on X defined his thesis as “we just need moar compute.” It’s bumper-sticker simple, but does summarize a reasonable approach to the future of AI. To train ever-more complex and accurate models, there just has to be more of everything: more data, more training and more run-time inference. All of those activities require more compute to process, and thus, more and more data centers. The winner of the AI race then is whoever has the most chips at the end of the night and can bankrupt everyone else at the table.
AI investors are rightfully paranoid in their search for durable moats in this fast-changing market. AI models surge ahead in relative performance and then fall behind, making it difficult to determine whether the market is winner-takes-all or whether multiple winners can secure niches or competitive advantages that sustain them. All model-building trade secrets eventually seep out, and engineering talent is hard to lock up, particularly in California where non-competes are unenforceable.
Conveniently for Altman, his approach with Stargate answers this need for a moat, and it’s basically, “I have more money than you.” It’s the lesson that Son of SoftBank apparently still believes, despite the exacting toll that thesis has had on the performance of the SoftBank Vision Fund since its debut in 2017 (which for the first time in years has turned to the black last quarter). Son, as Stargate’s chairman, seems to be doubling down on the cash-for-dominance strategy.
There’s two benefits to the capital moat. One is just the capital itself, which allows a company to flood the market at high burn while hiring as much relevant talent at the highest wages as possible to prevent other companies from competing. The second is that the lopsided balance sheet signals to investors not to dare to fund a competitor. Lightspeed is rumored to be funding Anthropic for $2 billion at $60 billion, and Altman is essentially looking at that petite pile of tokens at the table and rolling his eyes.
Yet, here’s the thing with capital moats: they really only work if you can monopolize a critical resource that halts competition. When Son was lavishly funding WeWork — his most infamous failure — the strategy was to monopolize all the key office buildings in major cities so that no co-working competitor could find a lease. For landlords, WeWork had this prodigious balance sheet and seemed a far safer tenant than any other hard-scrabble startup. So what went wrong? Outside of Covid-19 and fiscal mismanagement, the key strategic flaw is that WeWork couldn’t monopolize real estate. Supply was far more elastic than the company anticipated, and there were always more landlords who were willing to take WeWork’s rent money until the company’s ultimate collapse.
Can OpenAI monopolize compute? Here’s where the other key AI story this week comes in. DeepSeek, which I last covered in November, announced the launch of their R1 reasoning model with a paper on Arxiv and a full set of docs. In addition to being published under the very flexible open-source MIT license, the model’s performance efficiency gobsmacked many AI researchers. Nathan Lambert of Interconnects wrote that:
With o3, OpenAI is likely technically ahead, but it is not generally available nor will the weights be available anytime soon. This points to the first time since Stable Diffusion’s release that the most relevant and discussed AI model is released with a very friendly license. Looking back at the journey “open-source” AI has been on over the last 2.5 years, this is a surprising moment in time marked in the history books.
He notes that the efficiency and price difference is massive:
OpenAI was likely charging more for its model due to the costs of long-context serving and being the only model in town, but now o1’s pricing at $15 per million input tokens / $60 output looks out of place relative to R1’s pricing at $0.55 per million input tokens / $2.19 output (yes, o1-mini is cheaper at $3/$12 per million, but still almost a 10x difference). The price war that is coming for reasoning models will look like the Mixtral inference price war from 2023.
From the launch of DeepSeek’s V3 last year, we know that the company’s training costs are orders of magnitude less than OpenAI’s, which implies that compute can’t be monopolized (Lambert does have a better estimate of the “true” cost of training V3 which is higher than what’s been reported). DeepSeek’s public evidence endangers the entire narrative of OpenAI having a capital moat, and thus, its princely valuation.
DeepSeek is ultimately owned by High-Flyer Capital Management, a quantitive trading firm based in China. Are DeepSeek’s extremely competitive prices for AI reasoning purely a result of better model architecture and training, or is it attempting to demonstrate its own (more efficient) capital moat?
DeepSeek’s recent successes prove that more compute isn’t the only answer needed for better AI. The intellectual terrain of the artificial intelligence field remains shrouded by a fog of war. It’s not just about finding further optimizations to today’s transformers paradigm, but also searching for new mathematical foundations that can be orders of magnitude more efficient. It’s entirely possible that future developments may make $500 billion of data centers irrelevant.
For an historical analogy, look no further than search engines in the 1990s. As the web exponentially expanded, companies struggled to keep a handle on the scope of cyberspace. The answer from many companies was essentially “more compute,” transmuting the rich cauldron of capital of the dot-com boom into massive warrens of co-location facilities that dotted regions like the Bay Area. Then along came Google, PageRank, ultra-efficient sparse matrix math, MapReduce and a small set of other enabling technologies that radically improved the performance and efficiency of search.
Altman and Son are betting that hoarding dollars will protect them. They better hope no one else has a better idea.
Podcast: The on-going collapse of the global commons
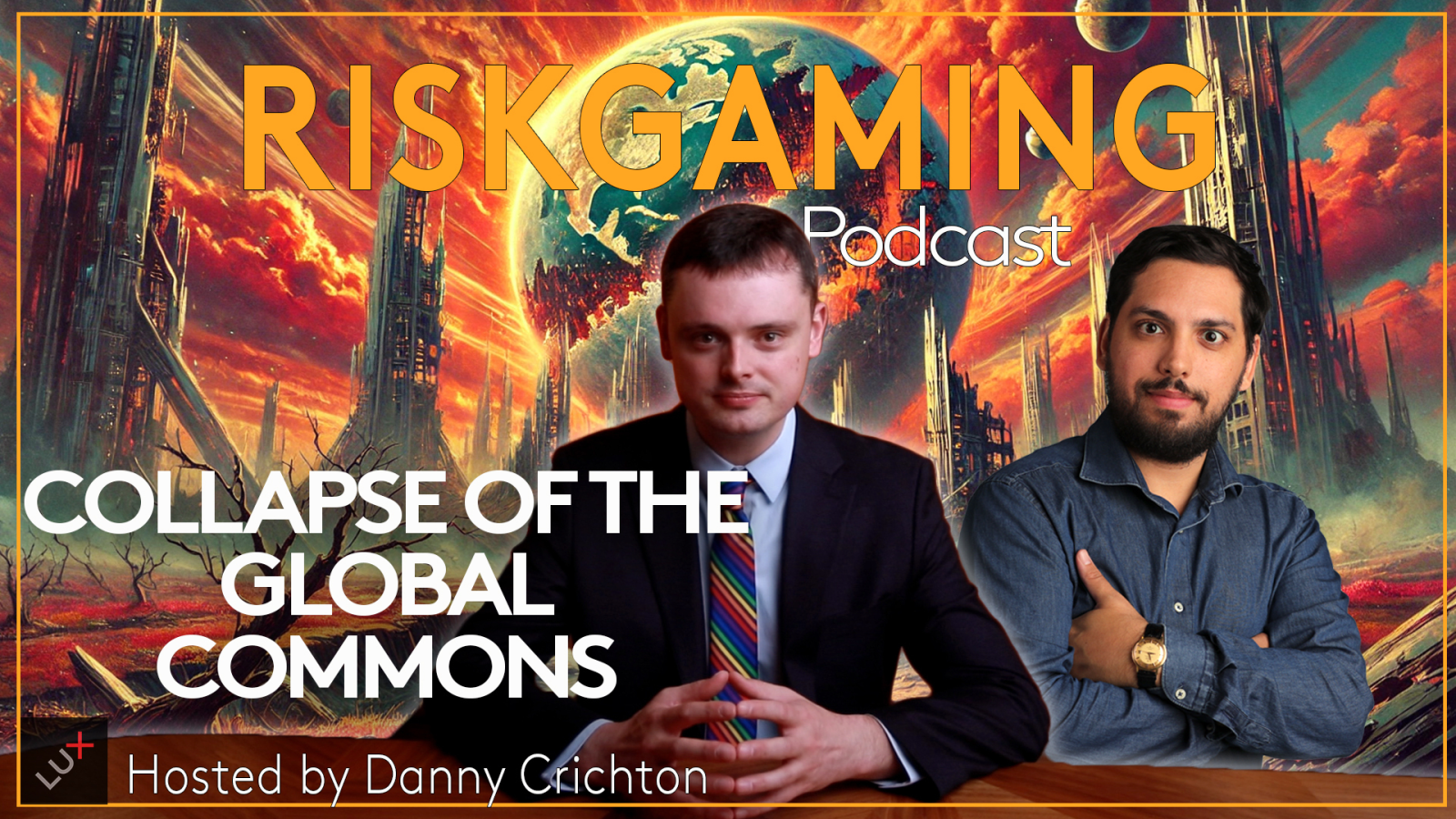
It’s been a bad few months (and years and decades) for the global commons. Chinese trawlers have repeatedly knocked out internet cables in international waters. Outer space is being militarized by Russia and others, threatening the demilitarized posture adopted by the Outer Space Treaty. Chinese hackers are using cyber weapons to infiltrate the U.S. Treasury through the Salt Typhoon hacks, while Antarctica is being explored by multiple militaries in contravention of the peace proposed in the Antarctic Treaty. Then there’s the decline of the information commons, where paywalls increasingly move critical news and data out of the reach of citizens.
In short, the global commons is losing primacy. Friend of the podcast Scott Bade (who last joined us in July) highlighted this theme for geopolitical strategy firm Eurasia Group’s annual Top Risks report, and we decided to follow up with our own Riskgaming conversation. So Laurence Pevsner and I teamed up to talk through the global commons and what’s endangering it.
We discuss the privatization and securitization of the commons, how post-World War II institutions are buckling under new pressures from rising powers, why technology is both helping and hurting, and finally, what America can do as a nation to stay open under threat.
🔊 Listen to “The on-going collapse of the global commons”
Lux Recommends
- Laurence enjoyed Michael Ignatieff’s essay in The Financial Times on “Canada, Trump and the new world order.” "Sigmund Freud’s theory — ‘the narcissism of minor differences’ — maintained that the smaller the real differences between two peoples, the larger these differences would loom in their identities. The differences between Canadians and Americans are so small that foreigners can’t tell them apart, and when Americans want to conceal their nationality from foreigners, which is often, they easily pass as Canadians. On the Canadian side, however, no one ever thinks our differences are minor.”
- I enjoyed Jordan Salama’s exploration of how Ecuadorian migrants use TikTok to project a far-rosier view of America than they actually live. “Affordable housing is nearly impossible to find, and many migrants end up renting single rooms in homes or apartments for about a thousand dollars a month, which they share with relatives or others to further defray the cost. The extreme stress regularly leads to alcohol abuse, domestic violence, and other issues. Such difficulties, however, are largely left out of the online picture; whenever sadness or nostalgia is expressed, it is vague and sweetly sentimental, conforming to an inspiring narrative of enduring temporary hardship to achieve future prosperity. More often, the struggle is not shown at all.”
- Laurence liked Joshua Rothman’s essay on "How Do You Know When a System Has Failed?” “Of all the intuitions we have about system failure, the most potent is probably that when a system fails, it fails totally. This is sometimes true: a car’s engine, for example, can burn out, failing completely. But, when that happens, the engine has actually been failing for a while. Its parts have been wearing down, its oil has been running low, its ignition timing has drifted, its catalytic converters have clogged. It just needed maintenance, which is normal. Systems need maintenance because they are always failing, just a little; that is, they are failing and functioning at the same time.”
- Although I discussed it above, Grace Isford really enjoyed Lambert’s look at how DeepSeek trained its R1 reasoning model. While technical, I found it illuminating on the state of the art for AI training, as well as a glimpse into how much we still don’t understand about which techniques work best.
- Finally, I am extremely late to this, but Michael Lewis’s profile “The Canary” in The Washington Post last year was extraordinary and a great transcon read. “There’s obviously something unusual about a person willing to spend a decade figuring out how to prevent roofs collapsing in longwall coal mines. ‘Why I find it so fascinating is a mystery to everyone I’ve ever met,’ said [Chris Marks]. ‘But I do.’ Most people capable of solving such a time-intensive technical problem would grow bored of it before they were done. ‘You have to be smart but not too smart to put in the years,’ as he put it. The federal government has long been a natural home for such characters: people with their noses buried in some particular problem from which they feel no need to look up. But once Chris had solved his particular technical problem, he had nothing to do but to look up.”
That’s it, folks. Have questions, comments, or ideas? This newsletter is sent from my email, so you can just click reply.