Why algorithms haven’t replaced human judgment in venture capital (yet)
Editor’s Note: Our Lux summer associate Dario Soatto wrote the following piece on decision-making and venture capital. This is his first solo column for the Riskgaming newsletter.
Say you devise an algorithm to predict whether someone will go to the movies tonight. On balance, this algorithm will outperform a human due to its ability to ignore psychological biases. But if you know that the individual broke their leg this morning, you can reliably predict that they won’t attend a movie, regardless of what the algorithm outputs. Paul Meehl, the clinical psychologist who pioneered research into the validity of expert predictions, referred to this scenario as the “broken leg rule”: if you have information of an extraordinary circumstance that an algorithm does not or cannot index on, then you can reliably outperform the algorithm.
When all legs are intact, however, human intuition often falls short.
Intuition refers to understanding something or making a decision without the need for conscious reasoning. Herbert Simon once defined expert intuition as “nothing more and nothing less than recognition.” That is, “The situation has provided a cue; this cue has given the expert access to information stored in memory, and the information provides the answer.”
At its best, expert intuition seems nothing short of magical — chess grandmasters spot checkmates at a glance, art experts identify fakes with a touch, and surgeons make timely interventions on a hunch. The psychologist Gary Klein tells the story of a firefighting commander who was hosing down a kitchen fire when he heard himself yell, “Let’s get out of here!” without realizing why. Shortly after, the floor collapsed, and the commander realized that the abnormally hot and quiet blaze had served as a cue that the heart of the fire had been in the basement underneath.
In contrast to tasks for which humans are hardwired — recognizing emotions on faces, for example — developing intuitive expertise for complex, professional applications like chess or firefighting takes years. Studies of chess masters, for example, have found that it takes at least 10,000 hours (that’s five hours per day for six years) of deliberate practice to attain the highest levels of performance—hence the oft-cited 10,000 hour rule popularized by Malcolm Gladwell. After these 10,000 hours of practice, the brain’s powerful associative machinery can map novel scenarios onto a rich database of experience and rapidly determine the best response, producing what we call expert intuition.
But this intuition can only develop if three conditions are met:
- The task/environment is sufficiently regular to be predictable
- There is an opportunity to perform prolonged practice to learn these regularities
- Feedback on performance is sufficiently fast and unambiguous to enable pattern recognition
The real world seldom conforms to these conditions. When operating in a domain with significant uncertainty and unpredictability (known as a low-validity environment), humans perform poorly: they index on irrelevant factors, fall prey to psychological biases, and often substitute the difficult question at hand (e.g. will this startup become a unicorn) with easier questions (e.g. do I like the founder’s personality). As a result, simple algorithms that predict outcomes by just aggregating relevant factors (e.g. frequency of lovemaking minus frequency of quarrels for predicting marital stability) often perform better than the relevant experts (e.g. marriage counselors).
Indeed, a robust body of literature has found that seemingly trivial algorithms, on balance, outperform the subjective judgments of trained professionals, whether school counselors predicting the grades of their students, physicians predicting life expectancy, bankers evaluating credit risk, judges discerning recidivism odds, sports pundits betting on football games or wine traders predicting future prices. As the late Daniel Kahneman wrote, “No exception has been convincingly documented.”
Editor’s Note: For more on Kahneman’s views, do listen to our Riskgaming podcast series “Risk, Bias and Decision Making,” a conversation between Kahneman, Annie Duke, Michael Maubaussin and our own Josh Wolfe.
Not only are we humans inept at making predictions in high-noise environments — we also struggle to distinguish between moments when our predictions are based on expertise versus those that are not. Regardless of whether we’ve accumulated enough high-quality experience to make expert judgments, our associative machinery produces intuitive answers to the questions we’re faced with — and it feels the same, creating an illusion of expertise.
Unfortunately, venture capital fits the bill for a low-validity environment: the information available when making investment decisions is extremely noisy, and investors often won’t know if they made a good investment until a decade down the line.
Even worse, decision-making in venture capital revolves around intuition. If you ask a venture capitalist how they decide which startups to invest in, they’ll most likely reply with something along the lines of “we look at the strength of the founding team, the quality of the business model/product, and the size of the market” — in fact, 95%, 74%, and 68% of surveyed venture capitalists index on those factors, respectively. But this response merely kicks the can down the road since the question of how venture capitalists judge teams, products and markets remains unanswered.
In contrast to private-equity firms, hedge funds, or asset managers, most venture-capital funds eschew the quantitative analyses traditionally employed in finance — there’s seldom cash flow models, comparative company analysis, ratios analysis, or anything that ends in “analysis” or “model.” Rather, venture capitalists rely on their own judgment: throughout their careers, whether in venture capital or otherwise, they develop heuristics regarding the types of founders, products, and markets that succeed and make decisions based on these heuristics.
In other words, they use intuition.
And intuition takes on an even bigger role in distinguishing between startups that merely pass muster and those worth investing in. A typical venture capital fund sees over 100 startups for every one that they choose to add to their portfolio; as a result, making an investment decision doesn’t just require judging that a startup has a high-power team and high-quality product in a high-opportunity market. Rather, there needs to be some extraordinary level of “conviction” that the company can achieve a unicorn exit, and the level of conviction must surpass that of the dozen other funds that passed on the investment. Oftentimes, determining whether the conviction is sufficient isn’t grounded in tangible observations and might not even be articulable — in other words, it’s based on intuition.
Are VCs doomed then? Fortunately not: outlier startups have a proverbial broken leg. The common denominator of successful startups isn’t some common factor identifiable algorithmically, but rather the fact that they each have some uncommon, extraordinary characteristic that is by nature outside of the distribution — and therefore difficult to systematically predict. This dynamic explains why the best investments often stem from personal relationships between investors and founders: the investors knew proprietary information that the founders had extraordinary potential that can’t be encapsulated by an algorithmic approach.
As a result, despite the pioneering efforts of firms like SignalFire, Correlation Ventures, and Ulu Ventures, quantitative venture investing hasn’t quite become the norm. If we had sufficient data on past startups and their outcomes, we could likely train a model that predicts which startups will enjoy venture-scale success; however, that data isn’t readily available. Due to the power law in venture capital, data on successful startups is inherently scarce, meaning that training models may require exponentially more data relative to asset classes where outliers matter less. Therefore, in the short-to-medium term, humans have an advantage over algorithms — and success isn’t merely based on luck.
In contrast to public equity markets, venture capital returns are both dispersed and persistent. Top performers and low performers in public equity trading generate similar returns (the 95th percentile returns 10% and the 5th percentile returns 5%), and year-by-year performance has almost zero correlation, suggesting that, in the vast majority of cases, public equity trading depends on luck, and much of the industry operates under an illusion of skill.
Venture capital returns, on the other hand, are highly dispersed (the 95th percentile returns 40% and the 5th percentile is in the red) and persistent (year-by-year returns have a 0.7 correlation). Therefore, the top venture capital funds must have secured a compelling, durable advantage that allows them to consistently generate outsized returns, whether in the form of outlier skill or network effects.
Nonetheless, the fallibility of expert intuition in low-validity environments holds important lessons for venture capitalists. Human intuition is easily fooled by a host of psychological biases, but studies have demonstrated that we can combat these biases by maintaining awareness of potential cognitive heuristics and focusing on objectively evaluating the characteristics that matter — that is, by focusing on the broken legs.
Podcast: Radical Uncertainty, Rapid Learning and the Success Equation for Catching Up
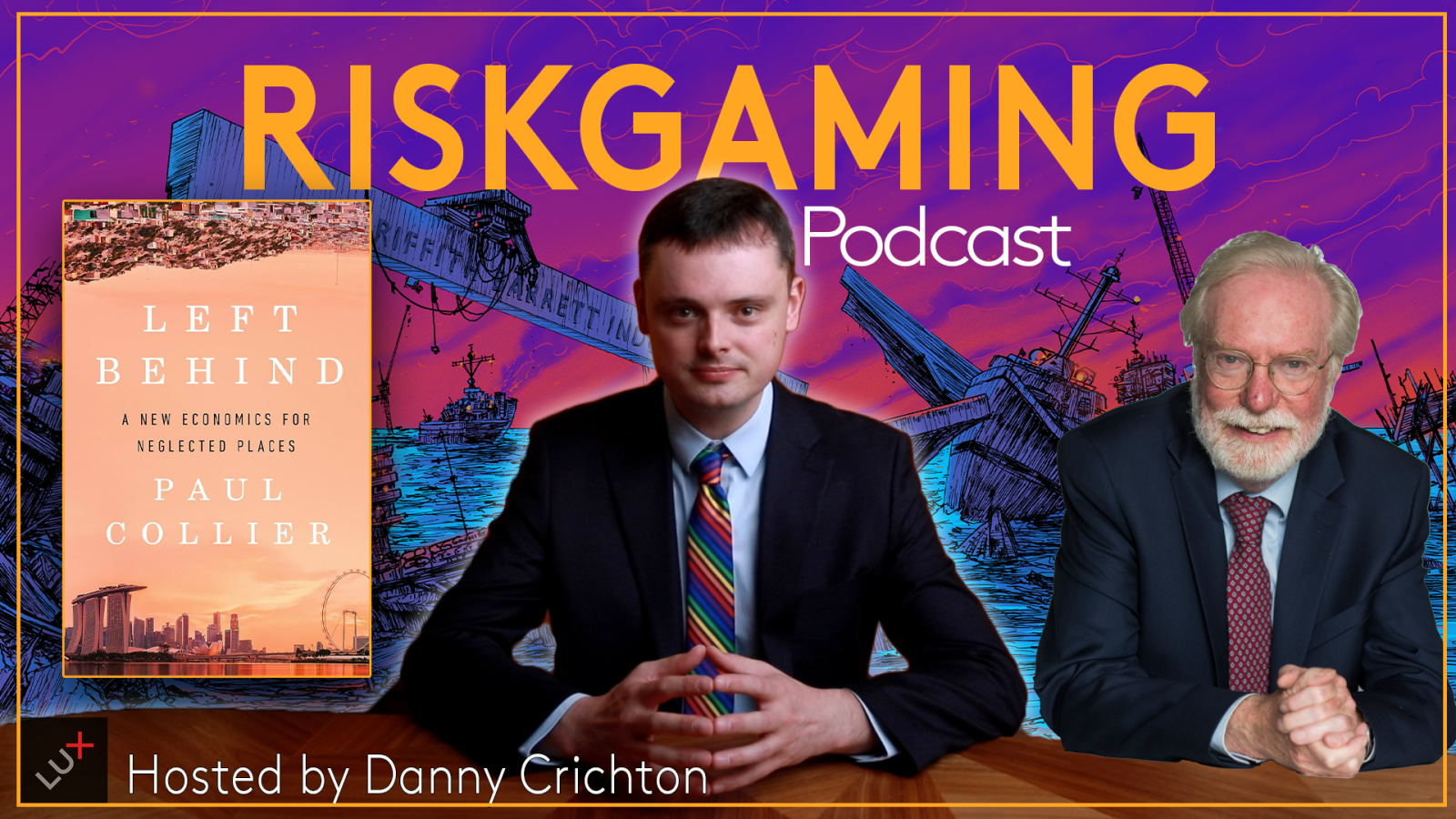
Global inequality has grown over the past two decades, concentrating an enormous amount of wealth and power on an elite number of individuals, cities, regions and nations all while stranding the vast masses to ignominy and penury. Yet, history is replete with examples of people and places that have caught up — and in some cases even surpassed — once foregone winners, begging the question: how should those left behind today work to catch up?
That’s the question that Paul Collier addresses in his new book, Left Behind: A New Economics for Neglected Places. Collier is a long-time development economist who has diligently brought the spotlight onto the most impoverished people in the world, calling attention to what he famously dubbed the “bottom billion.” With his new book, he explores why some places that were once terrifically wealthy — think Detroit in America — have fallen behind economically, and what steps are needed to overcome that stagnation.
We talk about the economic reversals of places around the world, why evolutionary economics and contributive justice offer new lenses on the problem, how addressing radical uncertainty through rapid learning suggests a path forward, and why global development institutions like the World Bank and the International Monetary Fund remain so recalcitrant in their approaches to aid, particularly in offering agency to those affected by their decisions.
🔊 Listen to “Radical Uncertainty, Rapid Learning and the Success Equation for Catching Up”
The Orthogonal Bet: Understanding Embodied Intelligence

In this episode, Lux’s scientist-in-residence Sam Arbesman speaks with Michael Levin, a biologist and the Vannevar Bush Professor at Tufts University. Michael’s work encompasses how information is processed in biology, the development of organismal structures, the field of Artificial Life, and much more.
Sam wanted to talk to Michael because of his pioneering research in these areas. Biology, as Michael’s work reveals, is far more complex than the mechanistic explanations often taught in school. For instance, the process of morphogenesis — how organisms develop their specific forms — challenges our understanding of computation in biology, and Michael is leading the way in this field. He has deeply explored concepts such as the relationship between hardware and software in biological systems, the process of morphogenesis, the idea of polycomputing, and even the notion of cognition in biology.
From his investigations into the regeneration process in planaria — a type of flatworm — to the creation of xenobots, a form of Artificial Life, Michael stands at the forefront of groundbreaking ideas in understanding how biology functions.
🔊 Listen to “How artificial life will power living computers”
Lux Recommends
- Our very own Deena Shakir’s new children’s book, Leena Mo, CEO, is exactly one month away from publication. It’s the inspiring story of Leena Mo, a young girl who decides one day to stop shoveling snow — and start building a robot named Helmy to do it for her. That’s just the first step on the journey of building a snow-blasting business that opens up the world before her.
- Lux summer associate Bradley Aka has posted an analysis on “Digital Twins In Healthcare.” “As you can imagine, these DTs have enormous applications and have recently made their way into healthcare. While these DTs are growing in complexity, many human health applications remain untwinned due to the complexity of modeling biological systems and human-food/drug interactions in vivo.”
- I enjoyed William T. Vollmann’s travelogue in Harper’s on the current and future meaning of the DMZ that splits the Korean Peninsula. “Link Farm was at present futile and therefore to a cynic almost ludicrous; but it just might be of practical use one day, a peace offering made more with sweat than with words. Paradoxically, the seven decades of separation might ultimately facilitate reconciliation, since most terrors and atrocities had occurred along the DMZ, which, as David habitually reiterated, was no focus of the attention of the majority…”
- Sam enjoyed Melanie Mitchell’s argument in Science on “The Turing Test and our shifting conceptions of intelligence.” “Although Turing’s original paper lacked specifics on how a test should be carried out, it was clear that the imitation game required three participants: a computer, a human foil, and a human judge. However, the meaning of the term ‘Turing Test’ in public discourse has evolved over the years into something considerably weaker: any interaction between a human and a computer in which the computer seems sufficiently humanlike.”
- Palmer Luckey, founder of Lux defensetech company Anduril, has been the subject of numerous profiles over the years, but none of them quite like Jeremy Stern’s in Tablet entitled “American Vulcan.” "But if he is perhaps the wildest misfit tech diva of his generation, with a torrid ambition and engineering prowess rivaled only by Elon Musk, Luckey is also, in a way Musk is not and cannot be, the product of something more familiar—the heir to a 100-year revolution in American society that made Southern California the techno-theological citadel of the Cold War, and a one-man bridge between the smoldering American past and an unknown future that may be arriving soon.”
- Roots of Progress Institute founder Jason Crawford joined us on the Riskgaming podcast last week, talking about his Techno-Humanist Manifesto. He just released chapter three, which Sam particularly enjoyed entitled “Ode to Man.” “Our rapid advancement is driven by another uniquely human faculty that deserves our reverence: we are the only species that can criticize ourselves. Biological evolution “learns” through births and deaths, but with the ability to theorize and criticize, to paraphrase Popper, our ideas can die in our stead. The ability to criticize is the ability to improve. Nature gives to every animal drives, but we are the only species that can choose to rise above them: not to kill whoever attacks us, not to devour any food in sight, not to father or bear as many children as we can. We criticize and improve even our own institutions: replacing monarchy with democracy, authority with science, witch hunts with jury trials.”
- Finally, I occasionally write on media with pieces like “Easternization of media” and “TechCrunch+ Termination.” Matthew Ball’s work is always original and filled with analytical nuance, and his latest deep dive into Roblox is no exception: “Roblox is Already the Biggest Game In The World. Why Can't It Make a Profit (And How Can It)?” “Run-rate spending on Roblox is over $3.8 billion (which likely exceeds that of any other game globally) and should pass $4 billion by the end of the year. Given these figures, it is no surprise that Roblox users are hyper-productive. In 2022, Roblox’s users designed 170,000 virtual clothing/accessory items and 15,000 virtual worlds each day (current figures are not known, though average daily users have grown 40% and the ease of creation has improved, as has the quality of the output). Over a hundred user-created worlds have been played over a billion times (and one has over 50 billion plays). Roblox also pays out a quarter of its revenue to its UGC developers, with more than $3 billion distributed since 2006 and a current annual run-rate distribution of more than $800 million.”
That’s it, folks. Have questions, comments, or ideas? This newsletter is sent from my email, so you can just click reply.