The emoji helping the machine learning community learn faster
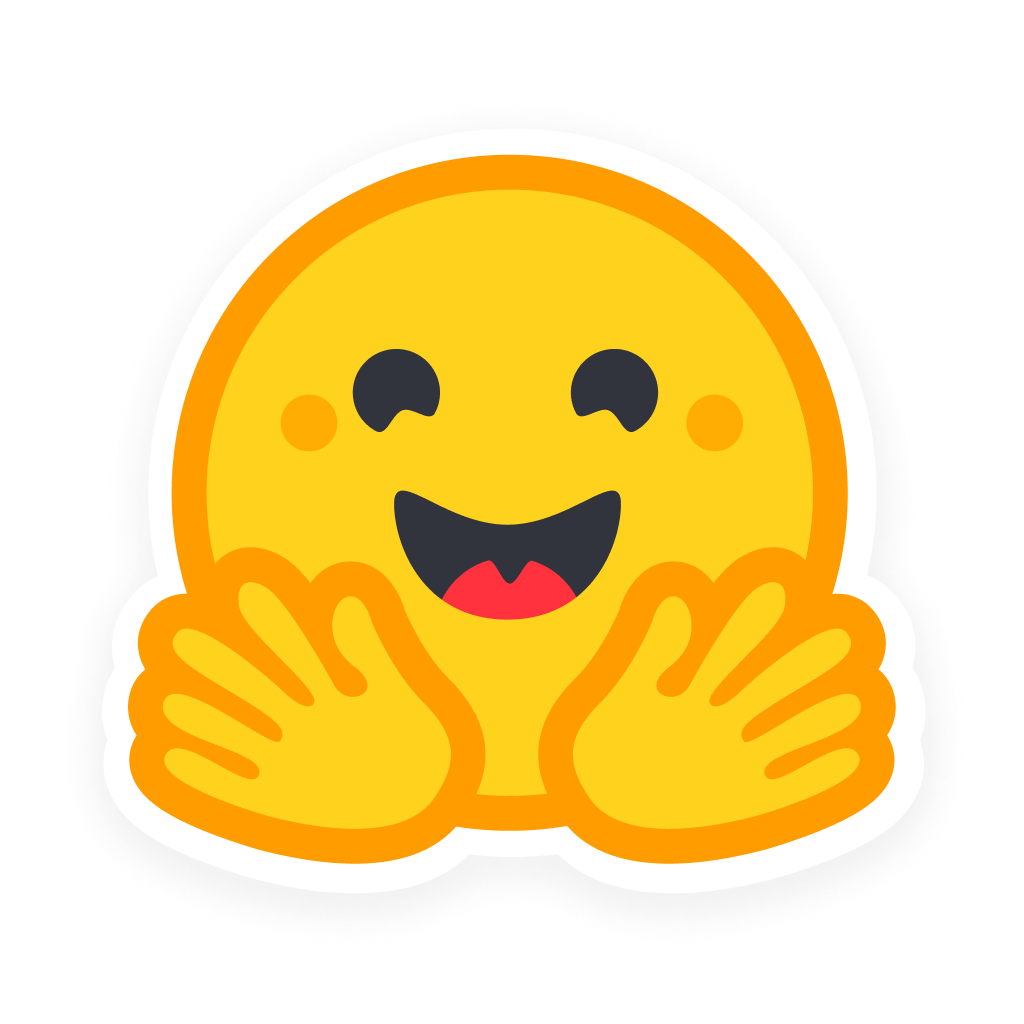
When it comes to turning sci-fi into sci-fact, few areas of computer science have had as complete a transition as machine learning. Once the bastion of 1960s speculative fiction novels, developers are now ubiquitously adding ML and artificial intelligence features to their apps and platforms. We search the web using information retrieval algorithms, find new music through intelligent recommender systems, autonomously drive based on computer vision neural networks, and invent whole new forms of media using high-powered language models.
Asimov’s dream is increasingly our reality.
This ubiquity isn’t an accident, but rather the collective output of legions of people who make it all possible. Machine learning engineers were once a small niche of developers perched in academic research centers. Now, their ranks have grown exponentially, and the average startup is increasingly making an ML engineer an early and critical hire.
Ubiquity doesn’t imply uniformity though. Machine learning is still a rapidly iterating field, where engineers specializing in different techniques, models, algorithms, and languages bring their combinatorial strengths together to synthesize the next generation of capabilities. It’s a community that is ferociously exploring the boundaries of what is possible with computing, and that forces each member to furiously track the improvements underlying the field.
While hundreds of companies offer an AI service, only one startup has made its focus on helping the learning community learn more effectively and share the best work globally. And it just so happens to be an emoji that is increasingly recognizable to every machine learning engineer the world over.
Hugging Face is just a few years old, but already, it has become as central to how AI/ML practitioners and researchers collaborate on building the future of the field as GitHub is to software developers. Building upon a set of APIs called Transformers that offered easy access to the best NLP machine learning models in a variety of programming languages, the startup founded by Clem Delangue, Julien Chaumond and Thomas Wolf now has 10,000 companies working on its platform while hosting 100,000 pre-trained models and more than 10,000 datasets. It’s also expanded from focusing just on NLP to areas as diverse as vision, speech, biology, chemistry and more.
We met Hugging Face several years ago and were enamored at the company’s potential to accelerate the already frenetic pace of evolution in machine learning. Its platform allows any individual or company to experiment with an ML model and remix it, all while offering better collaboration. It’s not often you can invest in a company with both a clear enterprise value and also an exponential societal value, and we pounced on the chance to work alongside Clem, Julien, Thomas and the whole HF team to finance and help them continue democratizing ML for everyone.
Today, Lux is announcing a continuation of our commitment, with a further investment in Hugging Face as part of its new $100 million Series C round, which was closed at a valuation of $2 billion. We remain as excited about the founders’ vision as when we first invested in 2019, and our board member Brandon Reeves will continue to work to protect and enhance the unique value that Hugging Face has brought the machine learning community.
Part of that unique value is the design of Hugging Face as an organization itself. The company — just like the ML community itself — is decentralized and global, with its largest office in Paris, a secondary hub in New York City and individuals including Clem spread throughout the world.
Second, and perhaps most importantly, Hugging Face has put addressing ethical concerns at the heart of its platform and ethos. Concerns about ethics in the AI and ML communities have become acute as these technologies have expanded to ever more critical applications. These technologies are no longer academic experiments, but instead power critical functions from lending underwriting to healthcare analytics. If a credit application for a mortgage is denied because the weighting of a model is skewed against a certain group of people, or an individual’s private health records are exposed through de-anonymization, then the ML community has failed in its duty to offer safe and trusted solutions to users.
Hugging Face has made addressing these concerns a mainstay concern in all of its product decisions and values, and the entirely team has compellingly shown a level of integrity needed as machine learning continues to expand rapidly this decade. User trust will ensure ML’s wide use and long-term positive acceptance.
The good news is that the learning community has more tools than ever to optimize their models and disseminate best practices. Hugging Face is designed to be a one-stop shop from planning and model building to training and execution, offering every engineer and researcher a seat at the table to improve the cutting edge of these technologies.
Take for example BigScience, an open consortium of 900 ML researchers who are collaborating to build a massive, 176 billion parameter multilingual neutral network language model. The consortium’s open practices mean that participants all around the world can improve facets of this model as it is trained at one of the largest supercomputers in the world in Paris. Meanwhile, Hugging Face will eventually host the final model when it finishes training later this year. It’s progress in science, and democratization of the cutting edge of machine learning.
We’re betting that Hugging Face won’t just continue to turn sci-fi into sci-fact, but will dramatically expand the capabilities of humanity to address the most urgent issues with the best computational techniques we have to offer.