It’s the final throes of cosmopolitan humanity
Distance creates difference. One of the key lessons from Alex Pentland’s pioneering work in “social physics” is that the depth of connection between two people declines precipitously as they move apart. That might not seem like a particularly keen insight, but the key question is how fast a drop over what distance? His research shows that even small distances can tremendously slow the spread of ideas. Switching to an office a few doors down a hallway can lower knowledge transmission by an order of magnitude, since serendipitous meetings are circumscribed by those additional few footsteps.
Indeed, the breathtaking diversity of human culture is a direct result of the historically impossible distances of the world. A limit on the speed of transportation and communications meant that different languages and civilizations could emerge just miles apart. France, today a mostly monolingual country, was home to more than a dozen languages ranging from Occitan to Angevin. Today outside of immigrant communities, just Basque appears to have the cultural scaffolding required to sustain its community of about a million speakers.
Distance is no longer just physical, of course. From the invention of the telegraph to the internet and now to large-language AI models, technology has regularly and rapidly narrowed the distances between people. Somewhat surprisingly, the rapid diffusion of these technologies has proven to be catastrophic for smaller language communities. The internet didn’t connect these communities tighter together; generally, smaller language communities were already tight-knit. Instead, it flooded these communities with global culture from dominant languages like English, overwhelming any cultural cultivation that might have resisted the tide.
Even so, many smaller languages hang on, driven by family ties, pride, culture, religion and other forces that allow them to continue to flourish or at least weather the global onslaught. The internet also facilitated groups like the Endangered Languages Project which maps and conducts research on how to protect these at-risk languages. Despite such efforts, dozens of languages have already gone extinct in just the last decade. UNESCO predicts that 3,000 languages could disappear by the end of the century, averaging a language roughly every two weeks.
Yet, even that dire prediction predates the sudden widespread popularity of large-language models since OpenAI’s ChatGPT debuted in November 2022. The key word to emphasize with large-language models is, of course, “large.” Training an LLM requires petabytes of text, audio and video available in a machine-readable format, an aggregate cultural output that only a few dozen languages have accumulated out of thousands spoken globally. For other languages, the LLM route is all but closed.
Last week, a group of researchers published IrokoBench on Arxiv, a new set of benchmarks for evaluating AI performance on 16 African languages that cover all regions of the continent. For example, Igbo, a language predominant in Nigeria, is spoken by roughly 31 million people, while Oromo, a language spoken by people in Ethiopia and Kenya, has roughly 45 million speakers. These are not near-dead languages, but rather thriving cultural communities.
The researchers found that leading LLMs like Meta’s open-source LLaMa 3, OpenAI’s GPT-4o and Anthropic’s Claude 3 Opus all struggled with knowledge reasoning in these languages. “On average, there is a significant performance gap between African languages and English (up to 45%) and French (up to 36%).” The researchers declare GPT-4o as the highest performance option, but they note that even it has made critical model tradeoffs. “It appears that GPT-4o’s enhancements for low-resource languages may negatively impact its performance on high-resource languages,” they wrote.
Fixing this performance gap is daunting. While these languages are spoken by tens of millions of people, a lack of literacy driven by poverty and slow economic development in their home countries limits the written text available to train LLMs. That immiseration also hinders the growth of digital infrastructure, preventing these cultures from expressing themselves online in a way that could be ingested into a dataset. Millions of people are speaking these languages every day, but none of that valuable data is being captured in a way that can be fed into an AI training algorithm.
While organizations like the Endangered Languages Project and UNESCO are building datasets for at-risk languages, what we’ve learned about AI model training so far is that we need breathtaking quantities of data to even hope to build a quality model. That’s why Nvidia’s stock has skyrocketed over the past few months: the whole world needs its chips to process as much data as companies can get their hands on.
That chasm between high-resource and low-resource languages is how the final mass extinction of differentiated culture arrives. It seems unlikely that boosting literacy for the next generation of speakers of low-resource languages while funding robust digital infrastructure will supply enough data to AI models in time before speakers themselves start to transition to more popular and dominant languages. Could Igbo and Oromo cross the dataset threshold required for having high-quality native LLMs? Short of a mathematical miracle in training AI with less data, the future prognosis is not positive.
LLMs will dominate the administration of more and more of the world, and it’s a recurring pattern of history that local and regional languages are ultimately subsumed by dominant administrative languages. Depending on one’s perspective for how fast AI models will replace human-to-human negotiation in commerce and government, these languages might be looking at just a few years to build their corpus — or begin the slow extinction process seen so often in the past.
While I have focused on languages with reasonably healthy communities of speakers, even the most spoken languages are becoming subsumed by English in artificial intelligence. English-centric models are dominant in all sectors of AI, driven by both the richness of the data available as well as the absolute supremacy of the United States when it comes to AI research. Most top models are trained heavily on English texts, and then additionally trained with other language materials in order to add multilingual capabilities. In its blog post announcing LLaMa 3, Meta writes that, “over 5% of the Llama 3 pretraining dataset consists of high-quality non-English data that covers over 30 languages.” Given that enormous skew, it’s unsurprising that some AI researchers show that models implicitly “think in English,” even when not specifically architected to do so.
In order to fix this intense bias toward English, researchers have taken two approaches. One is to train LLMs with a more balanced multilingual corpus (a category known as MLLMs). However, as a broad overview of MLLMs recently discussed, “MLLMs suffer from … the curse of multilinguality: more languages lead to better cross-lingual performance on low-resource languages up until a point, after which the overall performance of MLLMs on monolingual and cross-lingual benchmarks will decrease.” Similar to GPT-4o, there is a strict tradeoff between performance goals, a tradeoff that almost certainly won’t be in the favor of low-resource languages spoken by few.
Given the challenge of building performant MLLMs, that’s led to more and more national initiatives to build native single-language models with minimal English. Chinese Tiny LLM is an AI model built from the ground up to be Chinese native, using a massive Chinese text corpus complemented by a smaller dataset of English-language resources. CroissantLLM is doing the same with French, although the tokens still skew more heavily toward English. Lux’s own company Sakana AI is taking an East Asian-centric multilingual approach by centering around the ideographs in common between Chinese, Japanese and Mandarin-derived Korean vocabulary.
These are ambitious initiatives, but the underlying language trend is overwhelming. Human language offers the ultimate network effect: everyone wants to speak what everyone else can understand. The old joke goes that the world’s most popular language is bad English. As LLMs narrow the distance between cultures and commerce even further, the differences possible between communities are narrowing. Overwhelming incentives look set to wipe away all but a few languages by century’s end. The cosmopolitan humanism of the past millennia will homogenize to a single global culture. No amount of No, Non, Nein, 아니요, いいえ, 不, नहीं will stop the Yes to English.
Podcast: Why high-throughput bio research needs better tools immediately
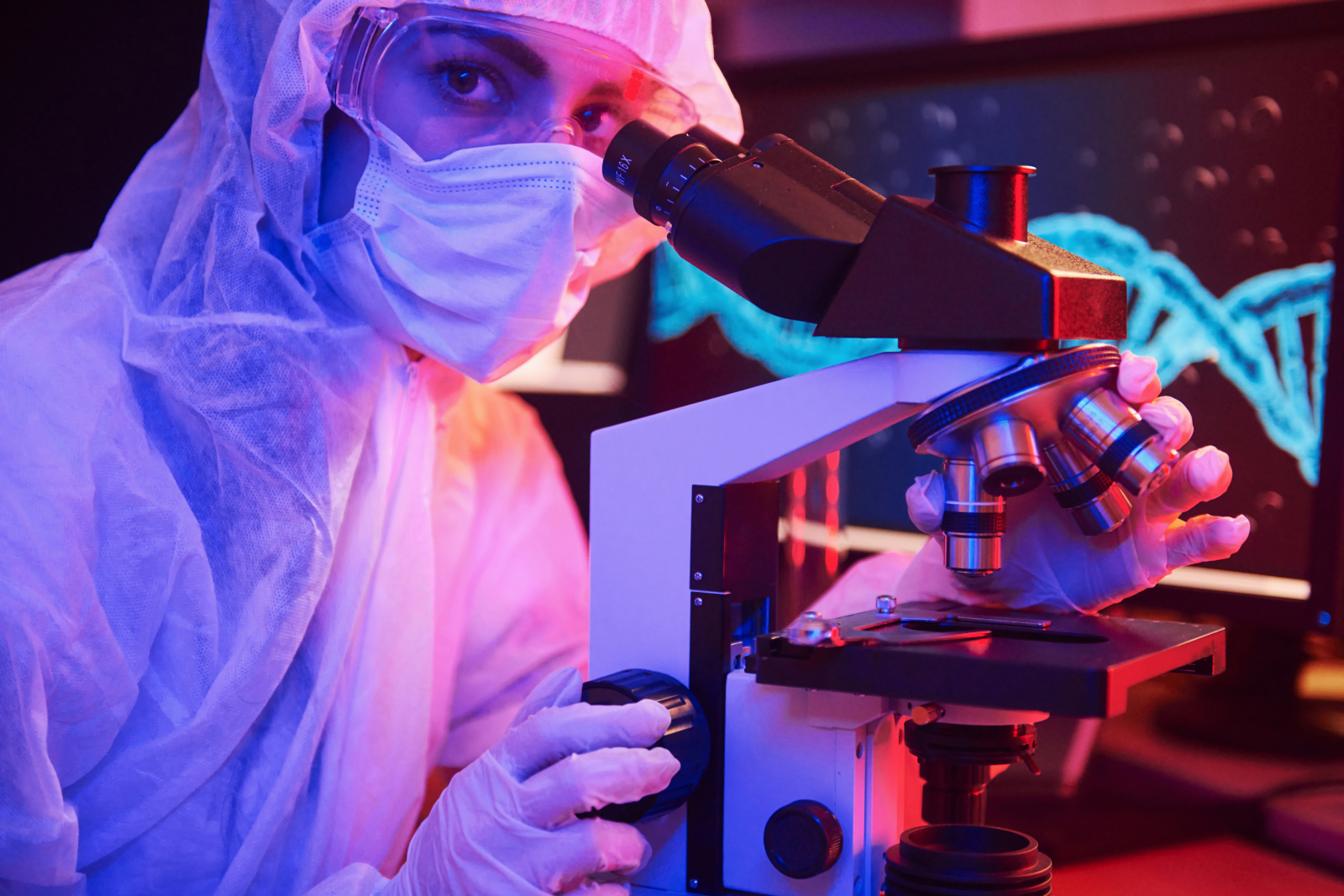
There have been data revolutions in most areas of human activity, and biological research is no exception. The rapidly shrinking cost of collecting data like DNA sequences means that there has been an exponential growth in the amount of data that bio researchers have at their disposal. Yet, most biologists still operate on top of general purpose cloud compute platforms, which don’t offer a native environment for them to engage in research at the cutting edge of the field.
On the Riskgaming podcast today, our own Tess van Stekelenburg interviews Alfredo Andere and Kenny Workman, the co-founders of LatchBio, who are on a quest to rapidly accelerate the progress of biology’s tooling. The big challenge — even for big pharma — is a lack of access to top-flight AI/ML developers in the ferocious talent wars they face against even bigger Big Tech companies. As Workman says, “They just don't have world's best machine learning talent … And then they're working with usually 5- to 10-year-old machine learning technology, except for a small handful of outliers.” LatchBio and other startups are pioneering new ways of delivering those tools to biologists, today.
In this episode, the trio discuss the changing data economy of biological research, the lack of infrastructure for conducting laboratory and clinical work, why AstraZeneca has improved its pharma output over the past decade, what the ground truth is around AI and bio, the flaws of open-source software, and finally, how academia and commercial research will fit together in the future.
Lux Recommends
- While we are on the subject of learning languages, another set of researchers has recorded more than 1,000 hours of meerkats in the wild, and then fed the audio into an AI model they are dubbing animal2vec. The hope is that AI may be able to find patterns in animal communication that are impossible for humans to grasp, but the challenge — once again — is lack of data. From the paper: “First, bioacoustics lacks datasets that are large enough to train transformer models with hundreds of millions of parameters and have enough fine-grain ground truth labels to enable effective finetuning. Second, bioacoustics has so far lacked a pretraining/finetuning training paradigm that takes advantage of the novelties in bioacoustics, like the high sparsity, noise corruption, and having raw waveforms as primary data format.”
- Our scientist-in-residence Sam Arbesman recommends an interview between former Riskgaming podcast guests and novelists Eliot Peper and Robin Sloane. “…I acknowledge the risk of scale, which is always: numbness. That's why dynamic range is important. Going big works better when you start small—when you zoom out step by step. Interestingly, I think video games, of all media, tend to do this best. Very often, you begin in darkness, on a little island of pixels. By the game's conclusion, whether it's an RPG or a civilization sim, you've opened up a vast world. It's thrilling! And I am bent on capturing some of that thrill for myself.”
- Antoine de Saint-Exupéry will forever be identified with The Little Prince (Le Petit Prince in French), but his non-fiction meditations on flying, adventure, and the meaning of life are just as compelling (and a bit more geared toward adult readers). I just read Wind, Sand and Stars and it’s just such an illuminating book on the human spirit. “Something in one’s heart takes fright, not at the thought of growing old, not at feeling one’s youth used up in this mineral universe, but at the thought that far away the whole world is ageing.”
- Rosecrans Baldwin at GQ has a story that I have hoped someone would write for a long time: Why Is Everyone on Steroids Now? “What patients sometimes fail to grasp, [Jessica Cho] says, is that testosterone doesn’t operate in isolation; the endocrine system is similar to an orchestra, where hormones work together for balance, not cacophony. She mentioned she also saw a lot of renal issues in men from consuming too much protein and too many supplements. ‘They’re taking crazy amounts of supplements and their kidneys are getting knocked out.’”
- Finally, Cameron Hudson on Why No One Will Save Sudan. “The most straightforward explanation is that there are too many crises in the world today for Sudan to pierce our consciousness. The war in Ukraine and the crisis in Gaza, where Western governments have far greater strategic interests at stake, are absorbing so much of the media’s attention, donor dollars, and policymaker time, that little is left to devote to Sudan. Officials euphemistically complain about a lack of ‘bandwidth.’”
That’s it, folks. Have questions, comments, or ideas? This newsletter is sent from my email, so you can just click reply.